Causation Entropy Boosting
- Jinlong
- Aug 13, 2024
- 2 min read
C. Chen, N. Chen, J.-L. Wu
Chaos 33, 083114 (2023)
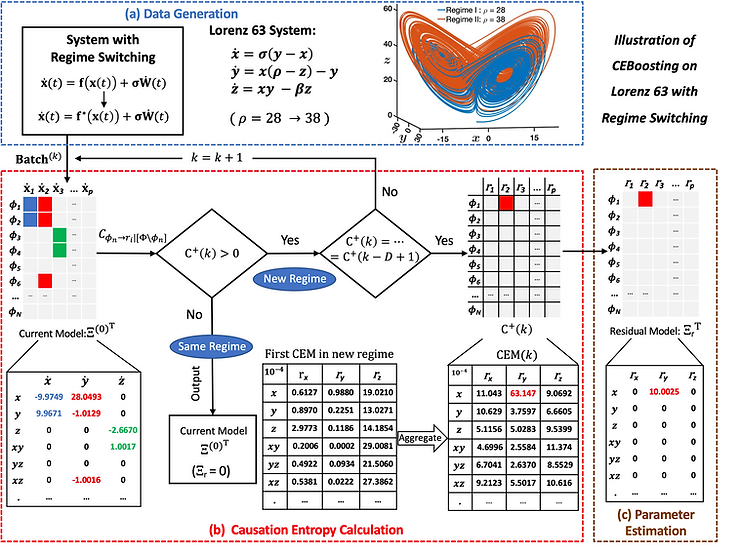
Online nonlinear system identification with sequential data has recently become important in many applications, e.g., extreme weather events, climate change, and autonomous systems.
In this work, we developed a causation entropy boosting (CEBoosting) framework to facilitate the detection of regime switching and the discovery of the dynamics associated with the new regime, via causality-enhanced online nonlinear system identification.
The causation entropy, which can be efficiently calculated, provides a logic value of each candidate function in a pre-determined library. For each sequential data batch, this framework calculates the causation entropy that evaluates the contribution of each function in a large set of candidate functions to the system dynamics.
The causation entropies based on multiple data batches are then aggregated to identify a few candidate functions that have significant impacts on the system dynamics. With the identified sparse set of functions, the framework further fits a model of the system dynamics.
We tested the proposed framework for complex systems with features including chaotic behavior, high dimensionality, intermittency and extreme events, and partial observations.
Using the Lorenz 96 model, it is shown that the causation entropy indicator can be efficiently calculated, and the method applies to moderately large dimensional systems. The CEBoosting algorithm is also adaptive to the situation with partial observations. It is shown via a stochastic parameterized model that the CEBoosting strategy can be combined with data assimilation to identify regime switching triggered by the unobserved latent processes. In addition, the CEBoosting method is applied to a nonlinear paradigm model for topographic mean flow interaction, demonstrating the online detection of regime switching in the presence of strong intermittency and extreme events.
Comments