Conditional Gaussian Neural Stochastic Differential Equation for Modeling Complex Systems and Data Assimilation
- Jinlong
- Aug 13, 2024
- 1 min read
C. Chen, N. Chen, J.-L. Wu
Computer Physics Communications, Volume 304, 2024,109302.
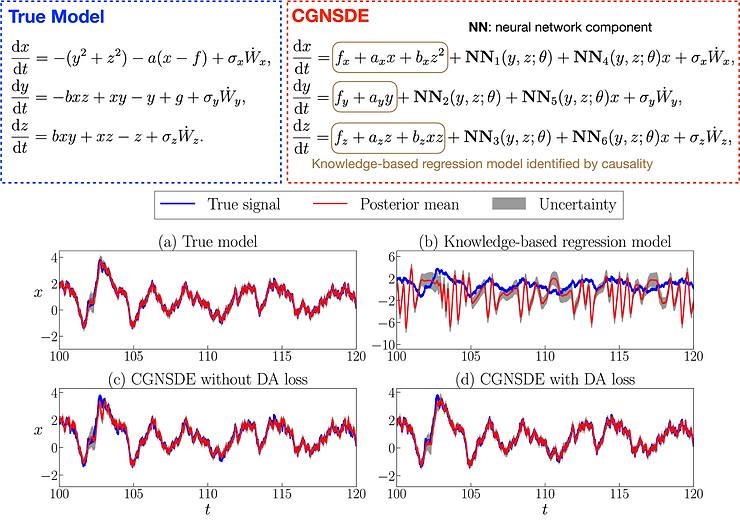
A new knowledge-based and machine learning hybrid modeling approach, called conditional Gaussian neural stochastic differential equation (CGNSDE), is developed to facilitate modeling complex dynamical systems and implementing analytic formulae of the associated data assimilation (DA).
In contrast to the standard neural network predictive models, the CGNSDE is designed to effectively tackle both forward prediction tasks and inverse state estimation problems.
The CGNSDE starts by exploiting a systematic causal inference via information theory to build a simple knowledge-based nonlinear model that nevertheless captures as much explainable physics as possible.
Then, neural networks are supplemented to the knowledge-based model in a specific way, which not only characterizes the remaining features that are challenging to model with simple forms but also advances the use of analytic formulae to efficiently compute the nonlinear DA solution.
These analytic formulae are used as an additional computationally affordable loss to train the neural networks that directly improve the DA accuracy. This DA loss function promotes the CGNSDE to capture the interactions between state variables and thus advances its modeling skills.
With the DA loss, the CGNSDE is more capable of estimating extreme events and quantifying the associated uncertainty.
Furthermore, crucial physical properties in many complex systems, such as the translate-invariant local dependence of state variables, can significantly simplify the neural network structures and facilitate the CGNSDE to be applied to high-dimensional systems. Numerical experiments based on chaotic systems with intermittency and strong non-Gaussian features indicate that the CGNSDE outperforms knowledge-based regression models, and the DA loss further enhances the modeling skills of the CGNSDE.
Comments